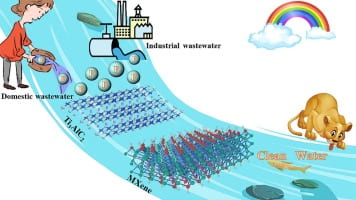
Huang, L., Sheng, L., Wan, K., Wang, M., Zhang, H., Yan, J., Liu, Y., Alhassan, S., Chen, Y., & Arulmani, S. R. B. (2024). The diversified mechanism of adsorption and electro-adsorption technologies by using Ti3AlC2 for removing fluoride. Desalination, 578, 117449.
This study explores the use of Ti3AlC2 for fluoride removal, highlighting its effectiveness in acidic conditions through adsorption and electro-adsorption mechanisms. It demonstrates that adsorption is more efficient at pH 2 than pH 1, with Ti3AlC2 undergoing multilayer sorption, chemisorption, and a partial transformation into MXene. Electro-adsorption outperforms sedimentation in fluoride removal at pH 1, with aluminum sites preferentially binding fluoride. The study combines experimental data and molecular modeling to detail Ti3AlC2’s fluoride removal mechanisms, offering insights into improving water treatment technologies. Learn more

Cao, G., Xing, H., Gui, H., Yao, C., Chen, Y., Chen, Y., & Li, X. (2024). Plasmonic quantum dots modulated nano-mineral toward photothermal reduction of CO2 coupled with biomass conversion. Nano Research, 1-12.
This research presents a novel approach for converting CO2 and biomass into chemicals using sunlight. Plasmonic bismuth (Bi) quantum dots were combined with phosphoric acid-modified attapulgite to catalyze the simultaneous reduction of CO2 and oxidation of benzyl alcohol. The catalyst’s design, leveraging Bi–O–Si bonds and surface plasmon resonance, enabled efficient electron transport and expanded light absorption, significantly improving reaction kinetics. The system achieved high rates of methane, carbon monoxide, and benzaldehyde production under solar irradiation, showcasing the potential for sustainable chemical synthesis. Learn more

Gao, J., Wahlen, A., Ju, C., Chen, Y., Lan, G., & Tong, Z. (2024). Reinforcement learning-based control for waste biorefining processes under uncertainty. Communications Engineering, 3(1), 38.
This study introduces a machine learning model to predict copper and poly(vinyl chloride) separation from cable waste, using an asynchronous-parallel recurrent neural network. Trained with six datasets across 3600 epochs, the model achieved high accuracy, with a mean-square error between 0.0015 and 0.0145. Sensitivity analysis identified the charged weight of cables and impact energy as key to efficiency. This approach could advance recycling technology by elucidating process mechanisms and optimizing performance. Learn more
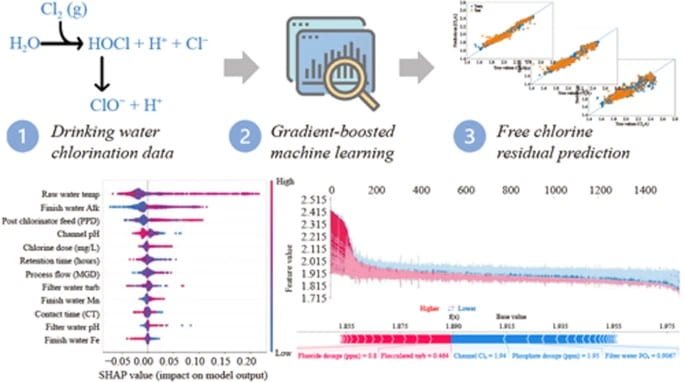
Helm, W., Zhong, S., Reid, E., Igou, T., & Chen, Y. (2024). Development of gradient boosting-assisted machine learning data-driven model for free chlorine residual prediction. Frontiers of Environmental Science & Engineering, 18(2), 17.
This study introduces a machine learning approach using CatBoost to forecast free chlorine residual (FCR) in drinking water treatment, leveraging a year of data from a Georgia plant. The best model achieved a 0.937 R^2 score, indicating high accuracy. Shapley’s additive explanations revealed non-intuitive, operational parameters significantly enhance prediction accuracy. This method offers a novel way to optimize chlorine dosing and improve water safety by integrating data-driven insights into conventional treatment processes. Learn more